Open-Source vs Closed-Source LLMs
Explore the impacts of open-source and closed-source large language models on AI innovation and accessibility.
In the rapidly evolving domain of artificial intelligence, the distinction between free-to-use closed-source and open-source large language models (LLMs) has significant implications for the future of technology and its accessibility. This analysis delves into the contrasting landscapes of these two types of LLMs, highlighting their unique roles and impacts in the AI industry.
Free-to-Use Closed-Source LLMs: Accessibility with Limitations
Closed-source LLMs like OpenAI's GPT-3 and Google's BERT and T5 offer formidable natural language processing and generation capabilities. These models, available through APIs, provide developers and businesses with powerful tools for various applications. GPT-3, renowned for its sophisticated language understanding, has revolutionised fields from content creation to customer service. Similarly, BERT and T5 have set new standards in contextual language understanding.
However, these models, while free to use initially (often under a trial or limited-tier model), are not open-source. While accessible to many users, the underlying code and training data are unavailable for modification or in-depth examination. This limits how users can customise or fully understand the model's internal workings.
The case for closed-source large language models (LLMs) is compelling, though it presents different advantages in artificial intelligence.
Focused Development and Specialisation: Closed-source LLMs are typically developed by specialised teams with significant resources, allowing for focused and sustained development efforts. This concentration of expertise and resources often results in highly sophisticated and efficient models, pushing the boundaries of what's possible in AI.
Controlled Quality and Consistency: A dedicated team manages the development and updates with closed-source models, ensuring consistent quality control. This can be particularly important for commercial applications where reliability and predictability are paramount.
Commercial Viability and Investment Return: The closed-source model allows companies to protect their intellectual property and ensure a return on investment. This is crucial for encouraging continued funding and research in AI, as it provides a financial incentive for companies to invest in long-term, high-risk research and development projects.
Enhanced Security and Privacy: Closed-source models can offer improved security, as the source code and training data are not publicly accessible. This can reduce the risk of malicious use of the technology and protect the proprietary data used in training the models.
Streamlined and Efficient Deployment: For businesses and users without AI expertise, closed-source LLMs offer a streamlined and user-friendly experience. These models are often delivered as part of a larger service package, reducing the complexity of deploying and maintaining AI systems.
Ethical and Responsible AI Development: Closed-source development can also allow for more controlled and responsible AI development. Companies can implement rigorous ethical guidelines and oversight during the development process, potentially reducing the risk of unintended biases or harmful uses of the technology.
Competitive Market Dynamics: Developing closed-source LLMs fosters a competitive market dynamic, continually driving companies to improve their offerings. This competition can lead to rapid advancements in the field, benefiting end-users with more sophisticated and capable AI tools.
Closed-source LLMs present a model of AI development that prioritises focused expertise, controlled quality, commercial viability, and security. While different from the open-source approach, this model plays a crucial role in the overall ecosystem of AI development, driving innovation and providing reliable, high-quality AI solutions for a wide range of applications.
Open-Source LLMs: Fostering Transparency and Innovation
On the other hand, open-source LLMs like Mistral AI's Mistral 7B, EleutherAI's GPT-Neo and GPT-J, and those from LLM360 offer a different kind of value. Released under licenses like Apache 2.0, these models provide complete access to their architecture and training data. Mistral 7B, for instance, stands out for its efficiency and capacity to compete with larger models while being freely available and modifiable.
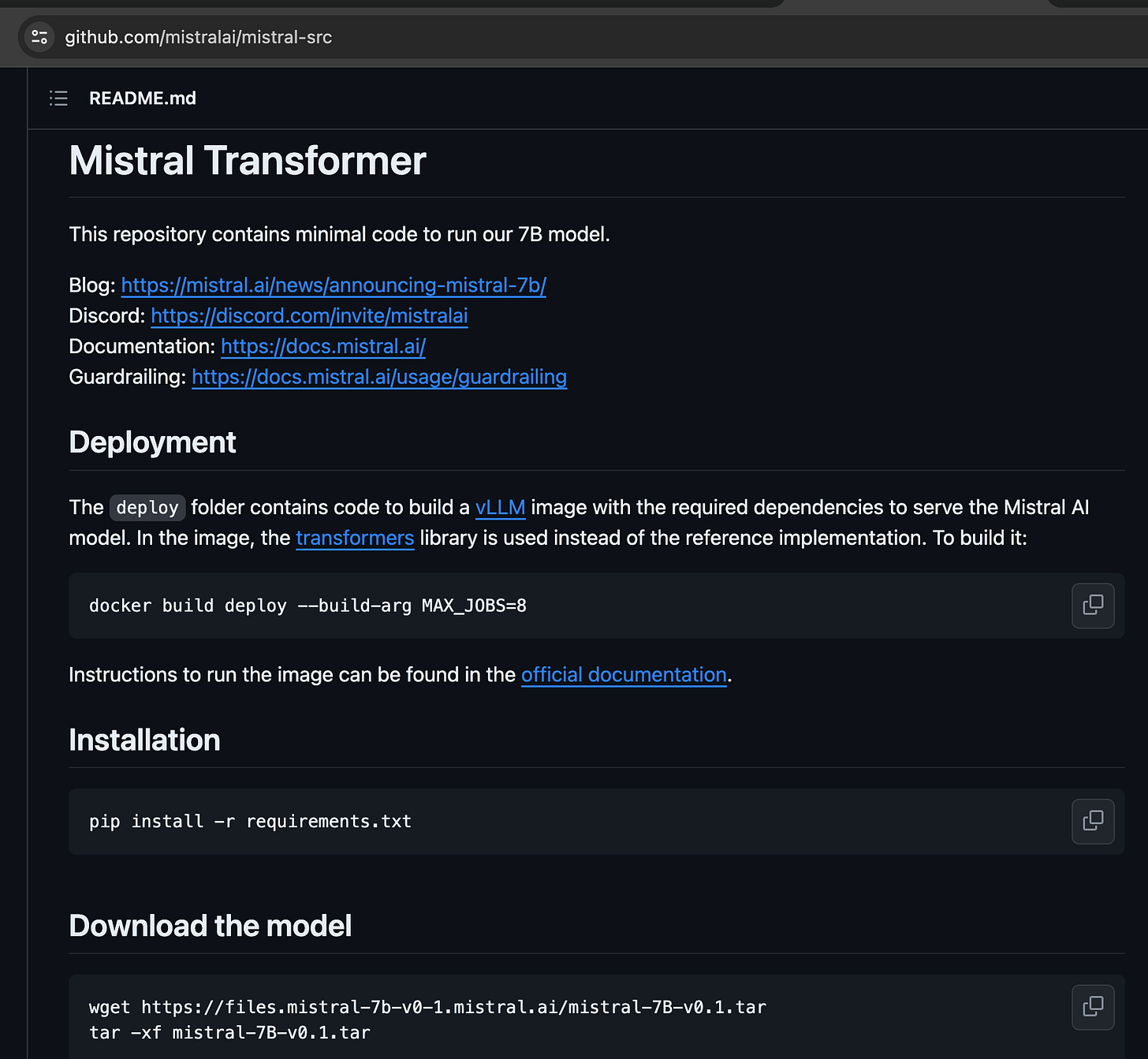
Only about a year after the launch of ChatGPT-3.5, I now have a GPT-3.5 class AI running on my home computer that is open source, free, reasonably fast, & doesn't require an Internet connection. -
,
EleutherAI's GPT-Neo and GPT-J have played a pivotal role in democratising AI technology, enabling researchers and developers to explore and innovate without the constraints of proprietary models. LLM360's initiative in releasing comprehensive open-source LLMs like AMBER and CRYSTALCODER further emphasises the importance of transparency and collaboration in AI development.
The democratisation of open-source large language models (LLMs) is an essential step in the evolution of artificial intelligence, with significant implications for cybersecurity, bug resolution, innovation, and accessibility.
Enhancing Cybersecurity through Transparency: Open-source LLMs contribute substantially to cybersecurity. By allowing a broader community of developers and researchers to access and review the code, vulnerabilities can be identified and rectified more rapidly and effectively. This collaborative approach to security is akin to a continuous peer-review process, where diverse perspectives and expertise converge to bolster the model's defences against potential cyber threats.
Prompt Bug Detection and Resolution: The open-source nature of LLMs facilitates quicker identification and resolution of bugs. A larger community of users experimenting with the model means that bugs, which might not be immediately evident to the original developers, are more likely to be discovered. This community-driven approach ensures continuous improvement and stability of the model, making it more robust and reliable over time.
Fostering Innovation and Customisation: Democratising access to LLMs spurs innovation by removing barriers to entry for researchers, startups, and independent developers. Open-source models can be adapted, modified, and built upon, allowing for a broader range of applications and innovations tailored to specific needs or industries. This flexibility is particularly beneficial for niche applications or underrepresented areas in AI research.
Educational and Research Advancements: Open-source LLMs are invaluable educational tools, enabling students and researchers to learn from real-world, sophisticated AI models. This access supports academic research and helps cultivate the next generation of AI talent, which is crucial for the field's continued growth.
Addressing Ethical and Bias Concerns: The open nature of these models also plays a crucial role in addressing ethical concerns and biases inherent in AI. A diverse group of contributors can help identify and mitigate biases, ensuring the models are more representative and fair. This is particularly important as LLMs are increasingly used in sensitive areas like healthcare, law, and recruitment.
Economic Implications: Open-source LLMs can level the playing field in the AI industry, allowing smaller and resource-constrained organisations to compete with larger entities. This can lead to a more diverse and competitive market, driving down costs and increasing the quality of AI products and services.
Global Collaboration and Community Building: Open-source models encourage global collaboration, transcending geographical and institutional boundaries. This fosters a sense of community and shared purpose, which is crucial for tackling global challenges through AI.
Democratising open-source LLMs is a multifaceted approach that strengthens the AI models regarding security and reliability and promotes inclusivity, ethical practices, and global collaboration in AI development.
Implications and Future Directions
The availability of free-to-use, closed-source and open-source LLMs is crucial for the growth and democratisation of AI technologies. While closed-source models offer potent tools readily available for various applications, they lack the transparency and flexibility of open-source models. Conversely, open-source LLMs encourage a more collaborative and innovative approach, allowing for more extensive customisation and understanding.
This blended ecosystem of LLMs is vital for fostering a diverse range of AI applications and research. It ensures that advanced AI capabilities are confined to large organisations with substantial resources and accessible to smaller entities and individual developers.
As the AI field continues to evolve, the interplay between these two models will shape the future of AI development, emphasising the need for accessibility and transparency in AI technologies. This dynamic landscape promises to bring more inclusive and innovative AI solutions, fundamentally altering how we interact and leverage artificial intelligence.